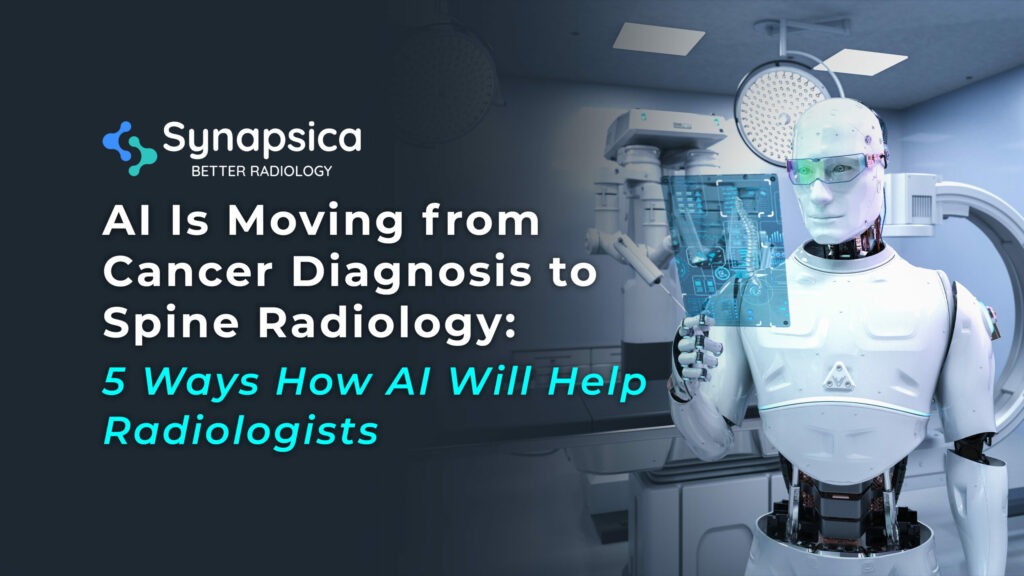
AI which is predominantly used in cancer detection is now entering spine radiology. Posited to be the upcoming global epidemic, spine problems are gradually transforming from a health problem to a social problem.
Spine problems can be the result of something as silly as lifting a heavy object or carrying heavy backpacks or falling accidentally from a bicycle. However, the major contributors to spine problems have been narrowed down to-
- Poor body postures
- Increased sedentariness in modern lifestyle
- Increased stress and anxiety
- Increased road accidents
One of the busiest countries in terms of road traffic, the U.S., records nearly 17,810 new spinal cord injuries (SCIs) every year. Around the globe, nearly 2,50,000 – 5,00,000 people suffer a spinal cord injury every year, mostly caused by preventable events like road traffic crashes, falls, etc.
The rampantly increasing spine problems and their socio-economic impact on the globe have made health tech firms and health providers experiment with the capabilities of AI in spine radiology.
→ Access Now: Radiology Software to Simplify Spine Analysis (Free Report)
And here’s how they believe AI will improve spine radiology and spine care:
- Determine the appropriate imaging test
- Optimize protocoling
- Creation of synthetic MR images from CT scans
- Quantitative analysis of spine images
- Improve TAT through automation
5 ways how AI-powered spine imaging will help radiologists
1. Determine the appropriate imaging test
The requests for cross-sectional imaging are increasing significantly in radiology. Hence, the determination of appropriateness criteria of imaging procedures (when to perform which procedure) is paramount. As recorded in the research paper, 32% of the MRI requests for the hip were believed inappropriate.
Also, uncomplicated low back pain accounts for a substantial amount of MRI overuse, leading to inessential healthcare expenditures and potential risks due to radiation exposure.
Given the long waiting queues for MRI tests due to increasing imaging tests, AI algorithms can help in automatically prioritizing urgent exams to avoid delayed diagnosis or treatment of time-critical conditions like spine tumors, hematoma, etc.
Machine learning and deep learning-based algorithms can help in automating and optimizing scheduling and protocoling. Machine learning algorithms can help in determining the most appropriate imaging examination for clinical conditions by evaluating clinical queries along with information ( like symptoms, previous imaging data, laboratory & pathology reports, physical examination findings, etc.) extracted from electronic medical records.
2. Optimize protocoling
A critical step in radiology workflow, protocoling helps in ensuring that the optimal step is performed for proper diagnosis. But protocoling is a time-intensive task that is mostly performed by radiology trainees or radiologists.
AI algorithms can help in optimizing protocoling. For instance, AI can automatically determine whether to include intravenous contrast in MRI examinations. In a research conducted to analyze AI’s performance in the determination of musculoskeletal MRI Protocol, a CNN (convolution neural networks) engineered to analyze whether MSK MRI scan should be performed as per usual tumor protocol exhibited 94.2% accuracy. AI algorithms can also help in selecting the pulse sequences and imaging planes for a protocol.
The AI-powered protocoling system can also help in avoiding risks during imaging tests by screening patient records for renal dysfunction, contrast allergy, pregnancy, presence of metallic foreign bodies, non-compatible implantable devices, etc.
3. Creation of synthetic MR images from CT scans
Artificial intelligence can help in expediting MRI examinations through undersampling and super-resolution. Using these techniques, high-quality images can be easily acquired without compromising diagnostic accuracy – which means MR images can be reconstructed with better quality and resolution, thus enabling automatic quality control and reducing the need for repeat examinations.
Even better, AI can help in creating synthetic MR images from CT scans. A study was conducted to evaluate the similarities between real and synthetic MRI images based on fat tissue, facet joint signal, disc signal, degree of disc protrusion, muscle, bone, thecal sac, degree of stenosis, and overall appearance, reported 80.2% similarity between the two images.
Generation of synthetic MR images from CT scans can highly benefit those patients who cannot undergo MRI.
4. Quantitative analysis of spine images
Despite the tech innovations in healthcare, image reading and reporting are performed in a subjective manner, which is one of the primary reasons for increased diagnostic errors and communication gaps in health systems and radiology practices. Though healthcare institutions are aware of the significance of quantitative analysis in reducing diagnostic and reporting errors, they are unable to perform quantitative analysis, due to the fast-increasing radiologist scarcity and the associated repercussions.
Also, due to the complex framework of the spine, it is easy to miss or overlook minute details in spine images, which may lead to unpleasant patient outcomes. Subsets of AI, such as machine learning and deep learning can help health systems perform quick and accurate image analysis quantitatively, enabling radiologists and physicians to quickly and correctly locate pathologies, monitor disease progression, calculate the age of the deformity, etc.
5. Improve TAT through automation
Radiologists perform numerous manual tasks on spine images before reading them for abnormalities. For instance, they check the quality of the scan, sort the normal images from the abnormal ones, mark the morphological points, locate and label spinal elements, prioritize and schedule cases, etc. Performing tasks manually contribute majorly to delayed diagnosis.
Well-trained AI algorithms can perform all these manual tasks, just the way a radiologist would do. Automating such tasks not just improves the speed of diagnosis, but also reduces reading errors, as most radiology errors are the result of time constraints.
AI in Spine Radiology – The Future Outlook
AI is changing spine radiology for the better. While we were concentrating on life-wrecking diseases like cancer, we were heedless of the physical and social repercussions of spine problems.
Also, since spine problems can co-occur, it is highly challenging for physicians and radiologists to pinpoint the root cause of the problem and it takes an extra pair of eyes to not miss out on the minor details hidden in the complex framework of the spine.
With the advent of AI, radiologists and physicians no longer have to be concerned about diagnostic and reporting errors due to subjective analysis, as AI can enable radiologists to do tough and time-consuming jobs like quantitative analysis and objective reporting within minutes, which is the need of the hour in radiology, let alone spine radiology.
Check out how Spindle and SpindleX, the AI-powered radiology software for spine MRIs and stress x-rays, are enabling instantaneous quantitative analysis and objective reporting. Click on the image below
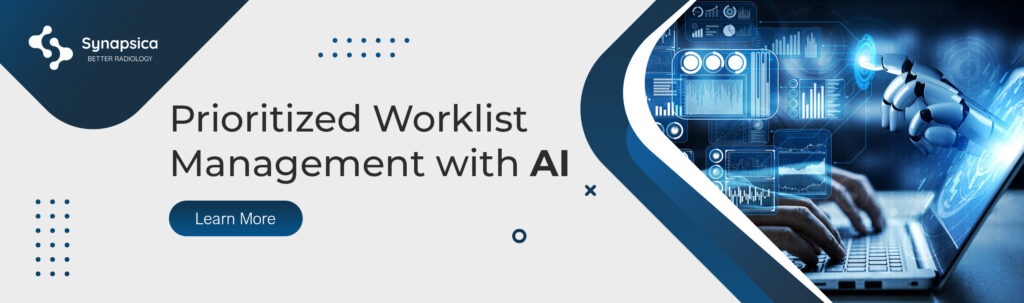